Genomes hold important clues to how species, and populations within species, adapt to their environments. Under rapid environmental change, an understanding of climate adaptation across a species range is important to both identifying vulnerable populations as well as populations that would thrive under future conditions and therefore be suitable for restoration efforts.
Although ecologists have a long history developing predictive models for species’ responses to climate change, it is only recently that models have been developed that incorporate genomic data into their predictions. These models are known as genomic forecasting models. Forecasts are often interpreted as degree of “genomic vulnerability” or the degree that fitness will decline under future climate change.
This perspective takes a foundational look at predicting species responses to climate change from different directions. From one direction, the question is asked: “What kind of prediction is needed for different applications?” First, the author shows that the type of prediction needed for restoration efforts (a prediction of which genotype will be the most productive in a particular habitat) is not the same type of prediction that is needed for estimating maladaptation to climate change (a prediction of productivity of a single genotype in different environments). Then, the author uses hypothetical and real data to show that these two types of predictions are not equivalent.
From another direction, the question is asked: “What do genomic forecasts predict?” Here, critical thinking is used to show that genomic forecasts likely predict which genotype will be the most productive in a particular habitat, due to the way that adaptive allele frequencies evolve on spatially heterogenous landscapes. The important take-home here is that a genomic forecast could predict the relative performance of genotypes grown in a common garden, but this does not necessarily validate the forecast for estimating the degree of maladaptation to climate change.
The perspective concludes with a discussion of how to design experiments to validate and improve predictions of species responses to climate change. Importantly, only experiments that are designed with multiple genotypes in multiple environments will provide the rigor necessary to validate predictions from genomic forecasting models.
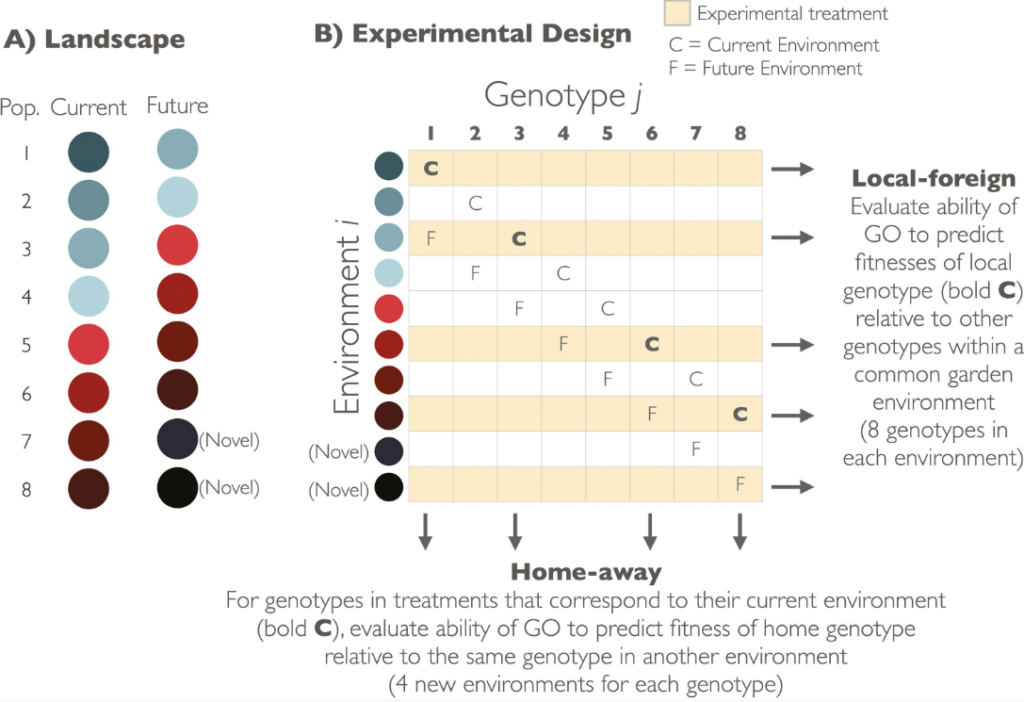
Figure and caption curtesy of Katie Lotterhos, and from the original paper.
In summary, this study integrates conceptual issues in local adaptation and conceptual issues in maladaptation to provide a foundational framework for constructing and interpreting predictive models. By integrating these different conceptual issues and including examples and code, the author offers a roadmap toward more robust and rigorous predictive models in ecology and evolution.